Major Updates for a Headstart in 2019
The year 2018 was a truly remarkable one here at Ximilar. These are the major news we would like to share with you.
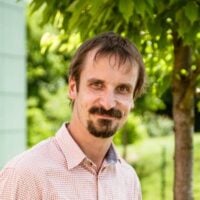
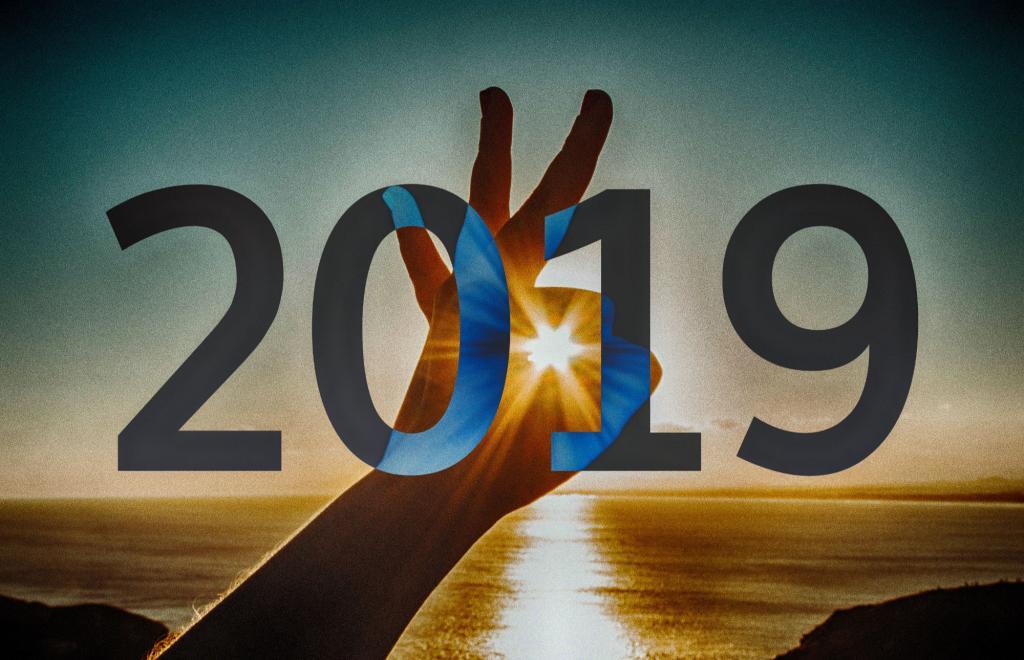
We have completely rebuilt the Vize system for image recognition and rebranded it as Ximilar App, expanded the team to cover more disciplines and most importantly we have grown our customer base. Besides that, we have worked hard on the product side of things to deliver even better service to all our existing customers, as there is fast development in the Computer Vision field every day.
It is visible that we are becoming experts in Fashion E-commerce and overall online commerce once the subject is image automation and data flow optimization. We either save significant expenses for our clients or improve their services for better conversion rates. Nevertheless, we are the backend guys, and we are not tangible that much to the end customer.
Redesigned Company Website
The redesign of the Ximilar website took a serious pile of man-days to create. The whole team got involved. And it is already paying off well. There is way more information about all the tools & features Ximilar offers. Spiced up with real-life use cases from many fields, including Fashion AI and E-commerce AI applications. There are examples and sample bits of code. And there is this blog to inform you about what is happening inside Ximilar.
We have also become an IBM Business Partner and expanded sales reach to Atlanta (USA), the United Kingdom and Asia. All that to be closer to you when you need a partner to help your business with the initial workings when embedding a robust Ximilar system inside your workflow.
Complex Documentation
This might seem like just a bunch of text at first look. But in reality, the documentation uncovers all the magic, all the possibilities that you get by using our universe of tools. Developers constantly update the docs, so you always have the most recent information at your fingertips. Making your life easier and supporting you in your busy day is our target.
Nevertheless, we are on email & live chat to help you anytime you require a helping hand.
New Feature: Precision/Recall table for Model
This is another critical feature to inspect the quality of your models, which should not be missed when developing a machine learning solution. We introduced a page with insight into your model in the Summer of 2018, and we are adding another advisory feature — Precision/Recall for each label. With that feature, you can verify exactly the level of reliability prediction for individual labels. The higher the value, the better your model succeeds in the prediction of these particular labels. The values have been detected from your training data, which is a random 20 % from all uploaded images in given labels.
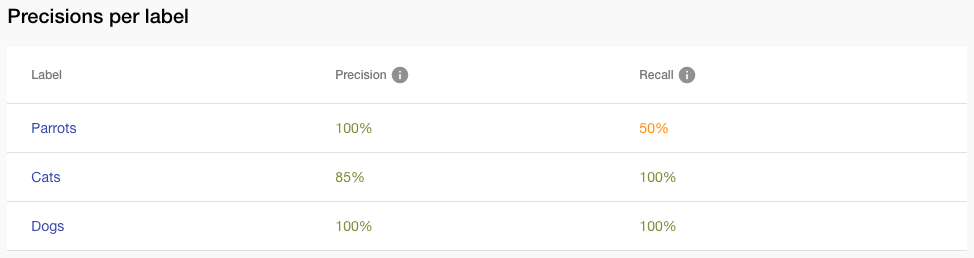
Example: the precision of label Cats means that 85% of images that were predicted by the model to be Cats are actually cats. Low precision numbers mean that the label is too broad – many images falsely get this label, and you should probably add more training images that are NOT Cats. On the other hand, the recall of 50 % of the label Parrots means that only 5 out of 10 images that actually are parrots were recognized by the model to be Parrots. Low recall numbers mean that the training data define this label as too narrow — this label is not recognized as often as it should be, and you should add more training images that ARE parrots.
New Feature: Advanced Settings for each Task
Many of our customers tell us that models from Ximilar Recognition provide better and faster results than models of our competitors (including the big players). Knowing your data, you can now further improve the reliability of your model by selecting the right checkboxes (horizontal flip, vertical flip, rotate 90). These settings are applied randomly to your images during the training (together with other modifications that are standard in machine learning). As a result, the trained model should then be invariant to the corresponding transformation (e.g., the recognition should be independent of the vertical flip of the image).
For example, many classifiers for microscope/medical data will benefit all three to be checked, as the important knowledge on the images can be rotated in all possible ways. The common practice for basic tasks, let’s say classifying houses, is to have just horizontal flip checked (default behaviour) as you probably do not want to classify a house upside down. You can experiment with the settings as you want and see what works best for your task.
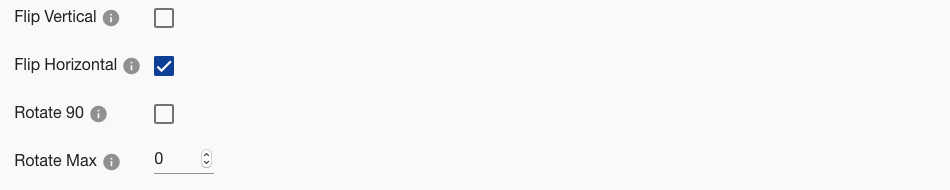
Improved & Updated Python Library
All the Ximilar Services are now behind the https://api.ximilar.com endpoint. That is why we made huge improvements to our Python library, which allows you to work with Ximilar Recognition (formerly Vize.ai), Dominant Colours, Generic Tagging & Fashion Tagging. The documentation, mentioned above, was changed to cover more knowledge, so the entire workflow of using the library is very straightforward. We still have further plans to expand this client by including more features and working with all possible endpoints.
More at https://gitlab.com/ximilar-public/ximilar-vize-api
Upcoming Features
And that is just the beginning of the year 2019. We already prepared many further features that are either requested by our customers or improve existing features to allow you to reach new horizons. These are just a few to give you a glimpse of what is coming:
- Image Tagging — or technically multi-label classification, where both the training images and the real data get more than one label/tag. A technique is often seen in stock photo agencies as photography keywords.
- Workspaces for Images and Tasks — To allow you to sort out your projects, should you have more than one.
- Improved User Interface — We are constantly iterating on the most common features.
Feel free to contact us and let us know what you are missing, or what would improve your system performance, speed or reliability. We are always on your side when it comes to reaching business targets or optimizing your expenses.
Tags & Themes
Related Articles
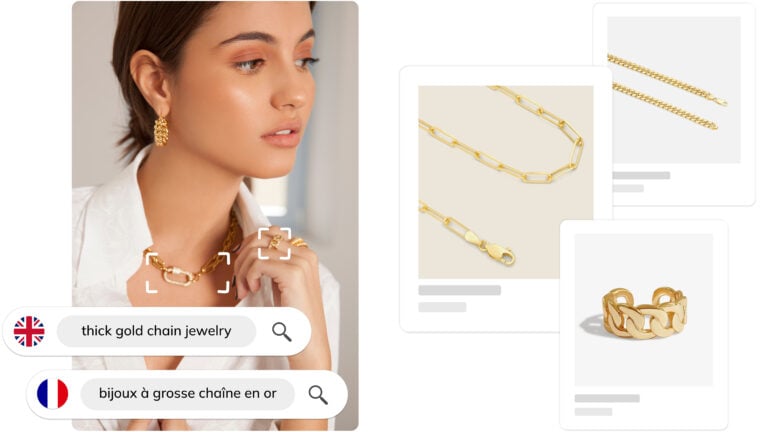
Ximilar Now Combines Visual and Text-to-Image Search
E-commerce retailers using our search engine now have access to multilingual text search as well.
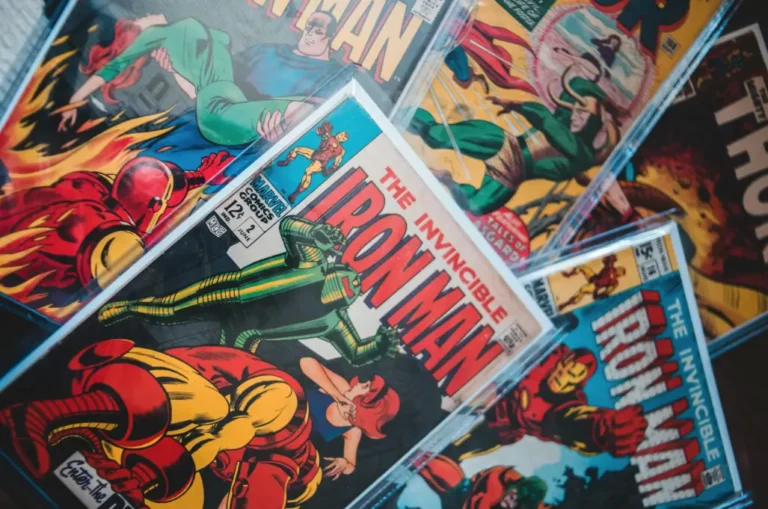
Identify Comic Books & Manga Via Online API
Get your own AI-powered comics and manga image recognition and search tool, accessible through REST API.
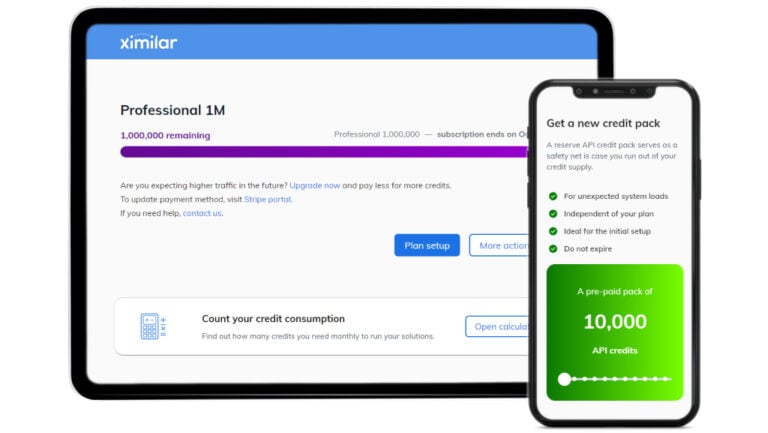
We Introduce Plan Overview & Advanced Plan Setup
Explore new features in our Ximilar App: streamlined Plan overview & Setup, Credit calculator, and API Credit pack pages.